US Army Has Developed 'DESOLATOR' To Protect Vehicles From Being Hacked
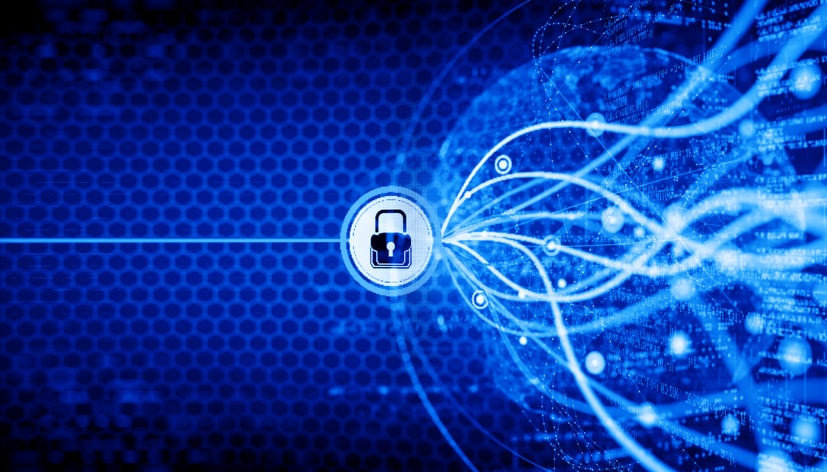
Highlights
- A technique called deep reinforcement learning has been used
- The technique is critical for securing its of in-vehicle networks
- It was designed by the US Army in partnership with academic institutions
Researchers in the US have developed a new machine learning tool called the DESOLATOR which has been designed to secure networks running inside a vehicle preventing them from being compromised and also not impacting the performance. The technology has been designed by the US Army in partnership with the Virginia Tech, the University of Queensland and Gwangju Institute of technology which will help optimise cybersecurity for moving vehicles which are often a target. DESOLATOR stands for deep reinforcement of learning based resource allocation and moving target defence deployment. It helps the in-vehicle network to identify the optimal IP shuffling frequency and bandwidth allocation to deliver effective long-term moving target defence.
"The idea is that it's hard to hit a moving target. If everything is static, the adversary can take their time looking at everything and choosing their targets. But if you shuffle the IP addresses fast enough, then the information assigned to the IP quickly becomes lost, and the adversary has to look for it again," said Dr Terrance Moore who is a mathematician with the US Army.
The team used an AI technique called deep reinforcement learning to gradually shape the behaviour of the algorithm. It used various reward functions like exposure time and the number of dropped packets to ensure DESOLATOR took both security and efficiency equally.
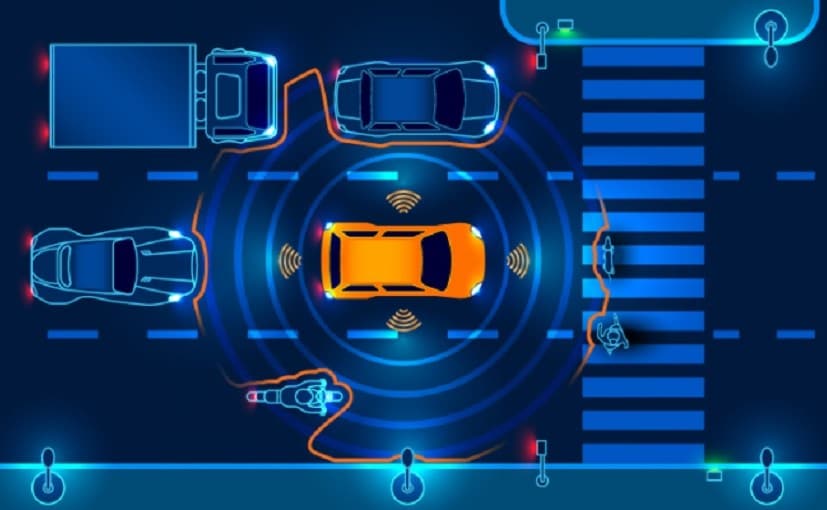
With cars being increasingly connected to the internet network security becomes more important
"Existing legacy in-vehicle networks are very efficient, but they weren't really designed with security in mind. Nowadays, there's a lot of research out there that looks solely at either enhancing performance or enhancing security. Looking at both performance and security is in itself a little rare, especially for in-vehicle networks," Dr Moore said.
It also is not limited to identifying then optimal IP shuffling frequency and bandwidth allocation. It is fundamentally a machine learning-based technique allowing goals within the problem space.
Another US Army computer scientist who is the programme lead, Dr Frederica Free Nelson claimed this level of fortification prioritised assets on the network which is a critical element of any kind of network protection.
"This ability to retool the technology is very valuable not only for extending the research but also marrying the capability to other cyber capabilities for optimal cybersecurity protection," Nelson said.
Last Updated on August 2, 2021
Latest News
- Home
- News
- Technology
- US Army Has Developed 'DESOLATOR' To Protect Vehicles From Being Hacked